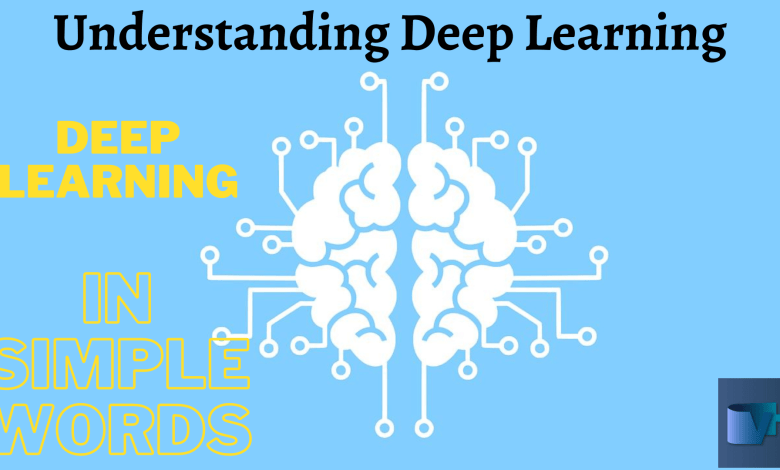
In recent years, the term Deep Learning has garnered considerable attention, promising remarkable breakthroughs in artificial intelligence (AI). It may sound complex, but fear not! In this article, we’ll unravel the mysteries of deep learning in layman terms, explaining what it is, how it works, and its real-world applications. So, let’s dive into the fascinating world of deep learning in simple words!
Deep learning is a field of AI that aims to mimic the human brain’s learning process. It involves creating computer algorithms called neural networks that can learn and make decisions from large amounts of data.
By learning from examples, deep learning algorithms can recognize patterns, make predictions, and perform tasks like image and speech recognition.
Examples of Deep Learning in the Real World include facial recognition technology, voice assistants like Siri or Alexa, and self-driving cars. Now, let’s explore the wonders of deep learning in simpler terms!
Deep Learning Definition
Deep learning is a cutting-edge field of AI that imitates the human brain’s ability to learn and make decisions. It revolves around creating computer algorithms called neural networks, which are inspired by the interconnected structure of brain cells. Just like our brain processes information, these algorithms learn from examples, gradually becoming smarter and capable of performing complex tasks.
Deep Learning Definition
Deep learning involves developing algorithms called neural networks that mimic how our brain learns and makes decisions. These networks consist of interconnected nodes, or artificial neurons, just like the cells in our brain.
They learn by analyzing vast amounts of data, gradually improving their performance. This allows deep learning algorithms to recognize patterns, make predictions, and perform tasks that were once considered challenging for computers.
By harnessing the power of deep learning, we can achieve remarkable advancements in fields like image and speech recognition, natural language processing, and even self-driving cars. It’s a fascinating field that enables machines to learn and think like human beings.
How Facebook Metaverse is Creating an entirely New World?
Deep Learning in Simple Terms
Let’s describe Deep Learning in simple words, it is like training a brain-inspired computer system to understand and analyze data on its own. Just as we learn from examples and gradually become better at tasks, deep learning algorithms learn from massive amounts of data to improve their performance.
Imagine teaching a computer to recognize different animals. Instead of manually telling it what features to look for in each animal, we show it a vast collection of images with labels. The deep learning algorithm starts by learning simple features like edges or colors and gradually builds up to complex ones like shapes and patterns. Eventually, it becomes proficient at recognizing animals by itself.
Deep learning is the technology behind many exciting applications we see today. From helping self-driving cars navigate roads safely to enabling virtual assistants to understand our commands, it’s making our lives easier and more efficient.
Deep Learning Algorithms
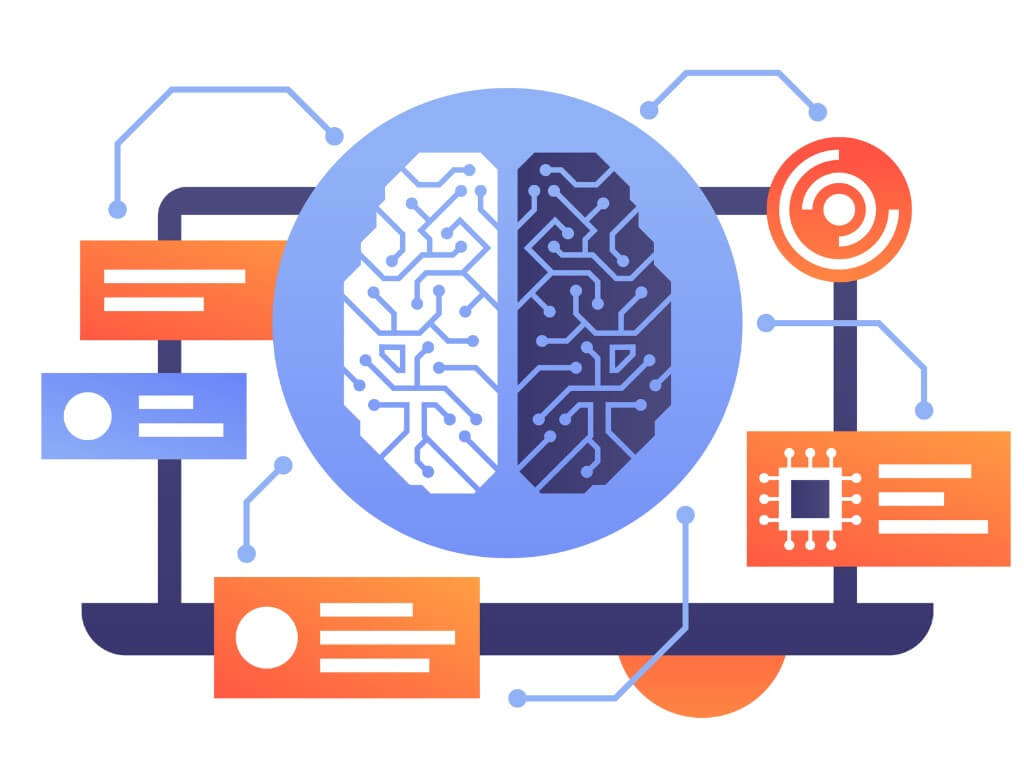
Deep learning Algorithms are the fundamental components of intelligent systems. They are composed of multiple layers of interconnected artificial neurons, forming what we call neural networks in Deep Learning.
These networks process information in a hierarchical manner, where each layer learns to extract increasingly abstract features from the input data. By analyzing enormous amounts of data, deep learning algorithms can identify complex patterns, make accurate predictions, and even generate new content, such as images, music, or text.
This hierarchical representation and ability to learn from data make deep learning algorithms powerful tools for solving a wide range of problems across various domains.
Deep Learning Examples
Here are some notable examples of deep learning applications:
Image Recognition:
Deep learning has revolutionized image recognition. For instance, it enables computers to accurately identify objects in photos or videos, such as recognizing cats, dogs, or even specific individuals.
Speech Recognition:
It powers voice assistants like Siri and Alexa, allowing them to understand and respond to our spoken commands. Deep Learning enables accurate speech recognition and natural language understanding.
Autonomous Vehicles:
This machine-learning model plays a crucial role in self-driving cars. By analyzing sensor data, deep learning algorithms can identify pedestrians, detect traffic signs, and make real-time decisions for safe driving.
Medical Diagnostics:
Deep learning aids in medical diagnosis by analyzing medical images like X-rays or MRIs. It can help detect abnormalities, assisting doctors in making accurate and timely diagnoses.
Natural Language Processing:
It is also used in language translation, sentiment analysis, chatbots, and language generation tasks, making interactions with computers more natural and effective.
Recommendation Systems:
Deep learning algorithms power personalized recommendation systems, such as those used by streaming platforms or online retailers, to suggest relevant content or products based on user preferences.
These examples illustrate the wide-ranging impact of deep learning across various industries and highlight its ability to tackle complex tasks with high accuracy.
AI and Deep Learning
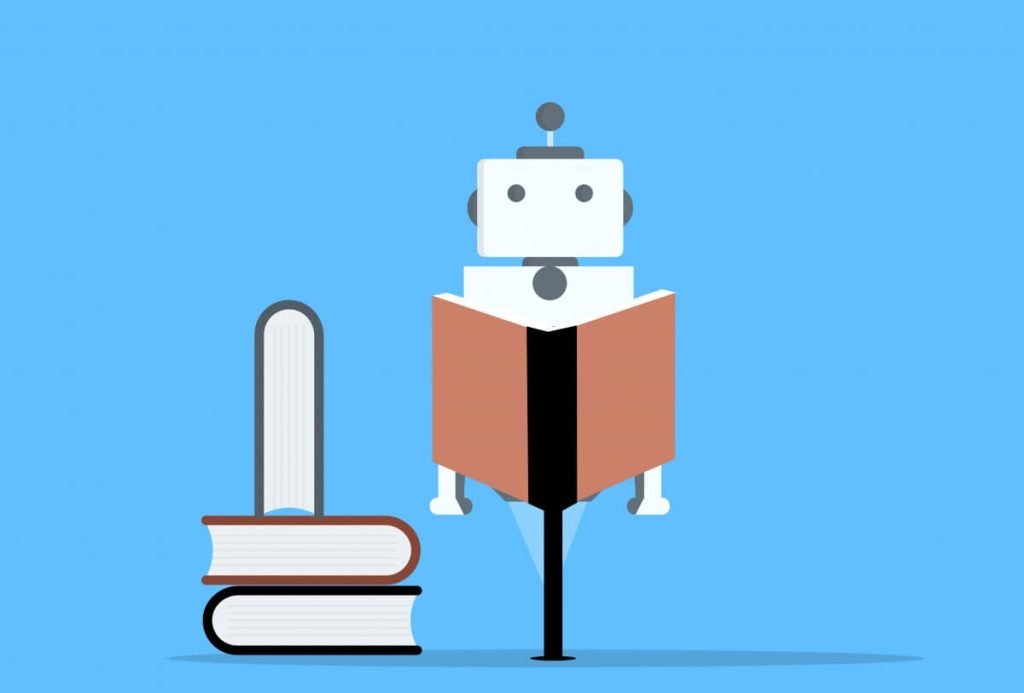
Artificial intelligence (AI) is a broader concept that encompasses various techniques, and deep learning in simple terms is one of its key components. Deep learning provides AI systems with the ability to learn from data automatically. It empowers AI to understand and interpret complex information, make predictions, and perform tasks with remarkable accuracy.
AI is a broad field that encompasses various techniques and methods aimed at creating intelligent machines. Deep learning is a specific approach within AI that focuses on training artificial neural networks to learn and make decisions from data, much like the human brain.
In simple words, deep learning is a powerful tool within the AI toolkit that enables machines to automatically learn and improve their performance by processing large amounts of data.
It’s like teaching a computer to learn from examples, allowing it to understand and interpret complex information, make predictions, and perform tasks with impressive accuracy.
So, deep learning is a key component of AI, empowering machines to become intelligent and perform tasks that were once considered challenging for computers.
Deep Learning vs. Machine Learning
Deep Learning and Machine Learning are closely related but distinct concepts within the field of artificial intelligence (AI).
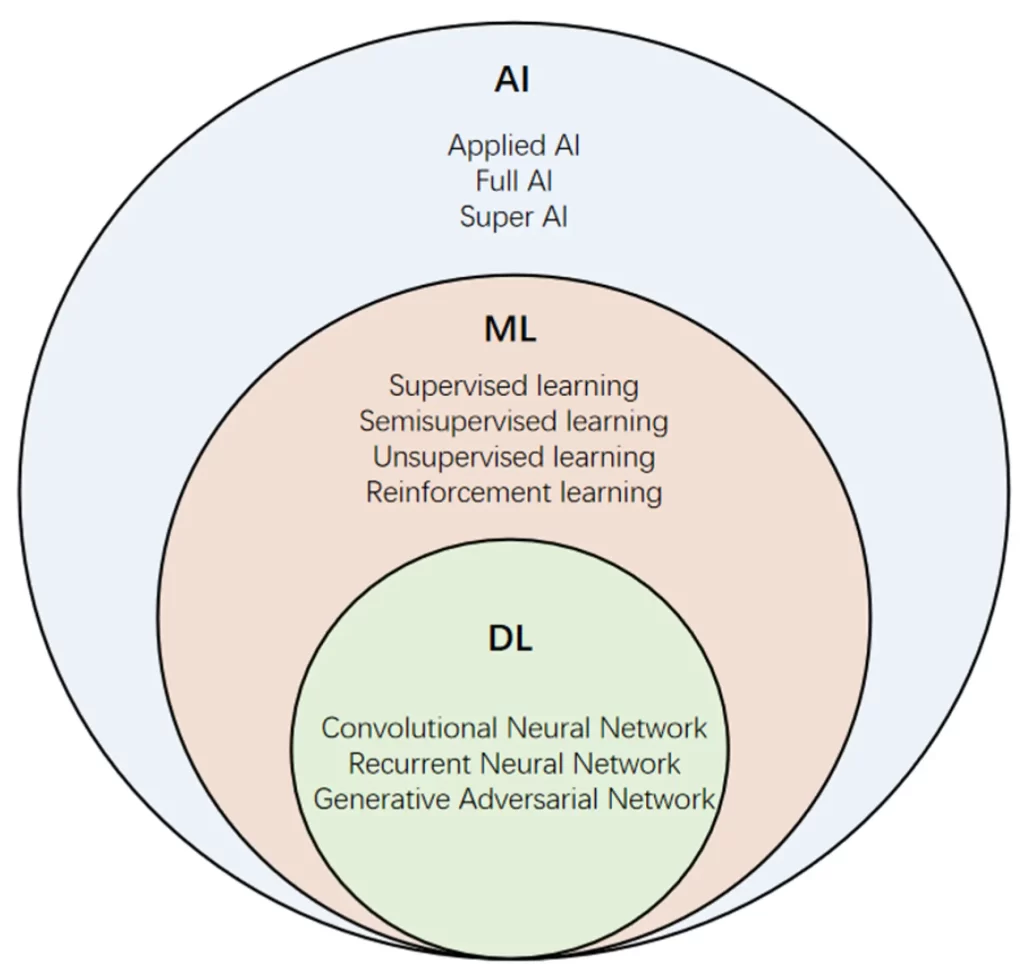
Machine Learning
Machine learning refers to a broader set of techniques where computers are trained to learn patterns from data and make predictions or decisions without being explicitly programmed.
The Machine Learning algorithms can be categorized into supervised, unsupervised, or reinforcement learning, depending on the type of training data and feedback they receive.
Deep Learning
On the other hand, Deep Learning in simple words is a specific subset of machine learning that focuses on training deep neural networks with multiple layers of interconnected artificial neurons.
These networks can automatically learn hierarchical representations of data, extracting increasingly abstract features as they progress through the layers.
Deep learning has shown remarkable success in areas such as image and speech recognition, natural language processing, and generative modeling.
While traditional machine learning algorithms require explicit feature engineering, deep learning algorithms can automatically learn relevant features from raw data. In other words, deep learning takes ML to the next level by autonomously discovering meaningful patterns and representations.
Is Israeli Missile Defense System is the Best in the World?
Deep Learning Techniques
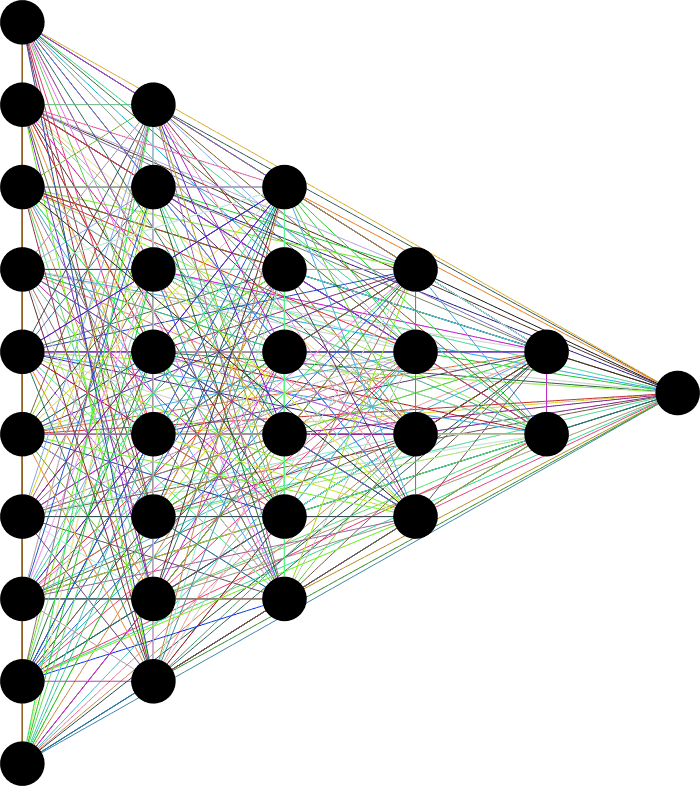
Within deep learning, several techniques and architectures enhance its performance and capabilities. Here are a few notable ones:
Convolutional Neural Networks (CNNs):
CNNs are used for image processing tasks. They learn to recognize patterns in images by analyzing small overlapping sections and sharing learned features. This helps in tasks like image classification and object detection.
Recurrent Neural Networks (RNNs):
RNNs are designed for sequential data like text or speech. They have a memory-like structure that enables them to retain information from previous steps. This helps in tasks such as language modeling, speech recognition, and machine translation.
Generative Adversarial Networks (GANs):
GANs consist of two networks—generator and discriminator—that compete against each other. The generator tries to create realistic data, while the discriminator tries to differentiate between real and generated data. GANs are used to generate new and realistic content, such as images or music.
Transfer Learning:
Transfer learning is about using knowledge gained from one task to help with another related task. It involves leveraging pre-trained models and fine-tuning them for specific tasks. This approach saves time and improves performance, especially when data is limited.
Reinforcement Learning (RL):
RL involves training an agent to make optimal decisions by receiving rewards or penalties in a given environment. Deep RL combines deep neural networks with RL algorithms, enabling the agent to learn complex behaviors. It has been successful in game playing and robotics.
These techniques are essential in deep learning and have led to significant advancements across various applications.
Conclusion
Deep learning, a fascinating field within AI, allows computers to learn and make decisions like humans. By developing algorithms that mimic the brain’s structure and function, deep learning achieves remarkable results across various domains, from image and speech recognition to medical diagnostics.
As deep learning continues to evolve, it opens up new possibilities and challenges in the world of artificial intelligence. Researchers are continuously pushing the boundaries to make AI systems smarter, more capable, and accessible to all.
So, this was an explained overview of deep learning in simple words, next time you interact with a voice assistant or marvel at the accuracy of image recognition, remember that deep learning is the powerhouse behind these innovations, enabling machines to learn, perceive, and make intelligent choices.
How Facebook Metaverse is Creating an entirely New World?
Frequently Asked Questions
What are deep learning examples?
Examples of deep learning include image recognition systems that can identify objects in photos, voice assistants like Siri or Alexa, self-driving cars that can navigate roads, language translation tools, and recommendation systems that suggest products or content based on user preferences.
What is the best way to explain deep learning?
Deep learning can be explained as a type of AI that imitates how our brain learns and makes decisions. It uses artificial neural networks with multiple layers to learn from data and recognize patterns. It’s like teaching a computer to learn from examples and become smarter over time.
Why is it called deep learning?
It is called deep learning because of the “depth” of the neural networks used. These networks have multiple layers of interconnected artificial neurons, which allow them to learn complex patterns and extract high-level features from data.
What are the benefits of deep learning?
Deep learning offers several benefits. It can handle large amounts of data, learn complex patterns, and make accurate predictions. It can automate tasks, improve efficiency, and provide valuable insights from diverse data sources. Deep learning also enables breakthroughs in areas like image and speech recognition, natural language processing, and autonomous systems.
How does deep learning work simply?
Deep learning works by training artificial neural networks with vast amounts of data. The networks learn to recognize patterns and make predictions by adjusting the connections between artificial neurons based on the input data. Through repeated training iterations, the networks improve their performance, becoming more accurate and capable of handling complex tasks.
What is the difference between deep learning and machine learning?
Machine learning is a broader field that includes various algorithms for training computers to learn from data. Deep learning is a subset of machine learning that specifically uses deep neural networks with multiple layers. Deep learning can automatically learn hierarchical representations of data, making it suitable for tasks with complex patterns.
What is the difference between neural network and deep learning?
A neural network refers to a network of artificial neurons interconnected with each other, which can learn from data. Deep learning, on the other hand, is a specific approach that uses neural networks with many layers to learn complex patterns and extract high-level features from data.
Is CNN deep learning?
Yes, Convolutional Neural Networks (CNNs) are a type of deep learning architecture. They are designed to process and analyze visual data, making them suitable for tasks like image recognition, object detection, and image generation.
What is the difference between deep learning and artificial intelligence?
Artificial intelligence (AI) is a broad field that encompasses various techniques to create intelligent systems. Deep learning is a specific approach within AI that focuses on training deep neural networks to learn from data. In simple terms, deep learning is a part of AI that enables machines to learn and make decisions from large amounts of data.
Which is better, machine learning or deep learning?
The choice between machine learning and deep learning depends on the task at hand. Machine learning is suitable for many applications and can provide effective results with less complexity. Deep learning, on the other hand, excels at handling complex patterns and tasks that require high-level representations. Each has its strengths and limitations, and the decision depends on the specific problem and available resources.
What is an example of a neural network?
An example of a neural network is a system that recognizes handwritten digits. By training on a large dataset of handwritten digit images and their corresponding labels, the neural network learns to identify digits accurately, even when presented with new, unseen examples.
What are the three domains of AI?
The three domains of AI are:
- Narrow AI: AI systems that are designed for specific tasks and have limited capabilities. Examples include voice assistants and recommendation systems.
- General AI: AI systems that possess human-like intelligence and can understand and perform tasks across different domains, exhibiting a broad range of cognitive abilities.
- Superintelligent AI: Hypothetical AI systems that surpass human intelligence and possess capabilities far beyond human comprehension.
Who invented deep learning?
Geoffrey Hinton, along with Yann LeCun and Yoshua Bengio, is considered one of the pioneers of deep learning. They made significant contributions to the development of deep neural networks and the advancement of deep learning algorithms. Geoffrey Hinton’s work on backpropagation and deep belief networks has been instrumental in shaping the field of deep learning.